Chuyển sang chế độ ngoại tuyến với ứng dụng Player FM !
A Closer Look at "Adversarial Robustness for Machine Learning" With Guest: Pin-Yu Chen
Manage episode 359226002 series 3461851
In this episode of The MLSecOps podcast, the co-hosts interview Pin-Yu Chen, Principal Research Scientist at IBM Research, about his book co-authored with Cho-Jui Hsieh, "Adversarial Robustness for Machine Learning." Chen explores the vulnerabilities of machine learning (ML) models to adversarial attacks and provides examples of how to enhance their robustness. The discussion delves into the difference between Trustworthy AI and Trustworthy ML, as well as the concept of LLM practical attacks, which take into account the practical constraints of an attacker. Chen also discusses security measures that can be taken to protect ML systems and emphasizes the importance of considering the entire model lifecycle in terms of security. Finally, the conversation concludes with a discussion on how businesses can justify the cost and value of implementing adversarial defense methods in their ML systems.
Thanks for checking out the MLSecOps Podcast! Get involved with the MLSecOps Community and find more resources at https://community.mlsecops.com.
Additional tools and resources to check out:
Protect AI Guardian: Zero Trust for ML Models
Recon: Automated Red Teaming for GenAI
Protect AI’s ML Security-Focused Open Source Tools
LLM Guard Open Source Security Toolkit for LLM Interactions
Huntr - The World's First AI/Machine Learning Bug Bounty Platform
48 tập
Manage episode 359226002 series 3461851
In this episode of The MLSecOps podcast, the co-hosts interview Pin-Yu Chen, Principal Research Scientist at IBM Research, about his book co-authored with Cho-Jui Hsieh, "Adversarial Robustness for Machine Learning." Chen explores the vulnerabilities of machine learning (ML) models to adversarial attacks and provides examples of how to enhance their robustness. The discussion delves into the difference between Trustworthy AI and Trustworthy ML, as well as the concept of LLM practical attacks, which take into account the practical constraints of an attacker. Chen also discusses security measures that can be taken to protect ML systems and emphasizes the importance of considering the entire model lifecycle in terms of security. Finally, the conversation concludes with a discussion on how businesses can justify the cost and value of implementing adversarial defense methods in their ML systems.
Thanks for checking out the MLSecOps Podcast! Get involved with the MLSecOps Community and find more resources at https://community.mlsecops.com.
Additional tools and resources to check out:
Protect AI Guardian: Zero Trust for ML Models
Recon: Automated Red Teaming for GenAI
Protect AI’s ML Security-Focused Open Source Tools
LLM Guard Open Source Security Toolkit for LLM Interactions
Huntr - The World's First AI/Machine Learning Bug Bounty Platform
48 tập
Tất cả các tập
×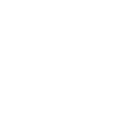
1 From Pickle Files to Polyglots: Hidden Risks in AI Supply Chains 41:21
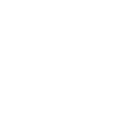
1 Rethinking AI Red Teaming: Lessons in Zero Trust and Model Protection 36:52
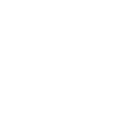
1 AI Security: Map It, Manage It, Master It 41:18
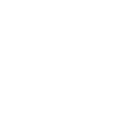
1 Agentic AI: Tackling Data, Security, and Compliance Risks 23:22
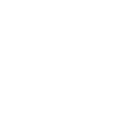
1 AI Vulnerabilities: ML Supply Chains to LLM and Agent Exploits 24:08
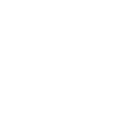
1 Implementing Enterprise AI Governance: Balancing Ethics, Innovation & Risk for Business Success 38:39
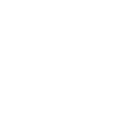
1 Unpacking Generative AI Red Teaming and Practical Security Solutions 51:53
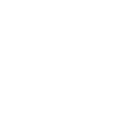
1 AI Security: Vulnerability Detection and Hidden Model File Risks 38:19
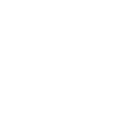
1 AI Governance Essentials: Empowering Procurement Teams to Navigate AI Risk 37:41
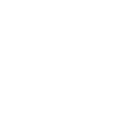
1 Crossroads: AI, Cybersecurity, and How to Prepare for What's Next 33:15
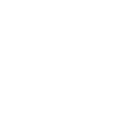
1 AI Beyond the Hype: Lessons from Cloud on Risk and Security 41:06
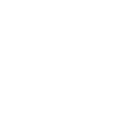
1 Generative AI Prompt Hacking and Its Impact on AI Security & Safety 31:59
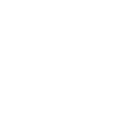
1 Exploring Generative AI Risk Assessment and Regulatory Compliance 37:37
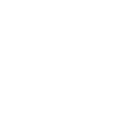
1 MLSecOps Culture: Considerations for AI Development and Security Teams 38:44
Chào mừng bạn đến với Player FM!
Player FM đang quét trang web để tìm các podcast chất lượng cao cho bạn thưởng thức ngay bây giờ. Đây là ứng dụng podcast tốt nhất và hoạt động trên Android, iPhone và web. Đăng ký để đồng bộ các theo dõi trên tất cả thiết bị.