521 subscribers
Chuyển sang chế độ ngoại tuyến với ứng dụng Player FM !
How Apache Iceberg and Flink Can Ease Developer Pain
Manage episode 439522425 series 75006
In the New Stack Makers episode, Adi Polak, Director, Advocacy and Developer Experience Engineering at Confluent discusses the operational and analytical estates in data infrastructure. The operational estate focuses on fast, low-latency event-driven applications, while the analytical estate handles long-running data crunching tasks. Challenges arise due to the "schema evolution" from upstream operational changes impacting downstream analytics, creating complexity for developers.
Apache Iceberg and Flink help mitigate these issues. Iceberg, a table format developed by Netflix, optimizes querying by managing file relationships within a data lake, reducing processing time and errors. It has been widely adopted by major companies like Airbnb and LinkedIn.
Apache Flink, a versatile data processing framework, is driving two key trends: shifting some batch processing tasks into stream processing and transitioning microservices into Flink streaming applications. This approach enhances system reliability, lowers latency, and meets customer demands for real-time data, like instant flight status updates. Together, Iceberg and Flink streamline data infrastructure, addressing developer pain points and improving efficiency.
Learn more from The New Stack about Apache Iceberg and Flink:
Unfreeze Apache Iceberg to Thaw Your Data Lakehouse
Apache Flink: 2023 Retrospective and Glimpse into the Future
4 Reasons Why Developers Should Use Apache Flink
Join our community of newsletter subscribers to stay on top of the news and at the top of your game.
888 tập
Manage episode 439522425 series 75006
In the New Stack Makers episode, Adi Polak, Director, Advocacy and Developer Experience Engineering at Confluent discusses the operational and analytical estates in data infrastructure. The operational estate focuses on fast, low-latency event-driven applications, while the analytical estate handles long-running data crunching tasks. Challenges arise due to the "schema evolution" from upstream operational changes impacting downstream analytics, creating complexity for developers.
Apache Iceberg and Flink help mitigate these issues. Iceberg, a table format developed by Netflix, optimizes querying by managing file relationships within a data lake, reducing processing time and errors. It has been widely adopted by major companies like Airbnb and LinkedIn.
Apache Flink, a versatile data processing framework, is driving two key trends: shifting some batch processing tasks into stream processing and transitioning microservices into Flink streaming applications. This approach enhances system reliability, lowers latency, and meets customer demands for real-time data, like instant flight status updates. Together, Iceberg and Flink streamline data infrastructure, addressing developer pain points and improving efficiency.
Learn more from The New Stack about Apache Iceberg and Flink:
Unfreeze Apache Iceberg to Thaw Your Data Lakehouse
Apache Flink: 2023 Retrospective and Glimpse into the Future
4 Reasons Why Developers Should Use Apache Flink
Join our community of newsletter subscribers to stay on top of the news and at the top of your game.
888 tập
Alla avsnitt
×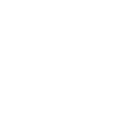
1 OpenSearch: What’s Next for the Search and Analytics Suite? 20:10
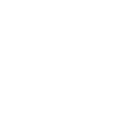
1 Kong’s AI Gateway Aims to Make Building with AI Easier 21:05
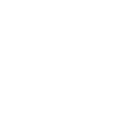
1 What’s the Future of Platform Engineering? 26:44
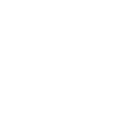
1 AI Agents are Dumb Robots, Calling LLMs 28:31
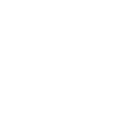
1 How Generative AI Is Reshaping the SDLC 21:42
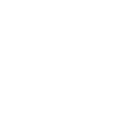
1 OAuth Works for AI Agents but Scaling is Another Question 25:36
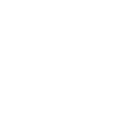
1 LLMs and AI Agents Evolving Like Programming Languages 28:08
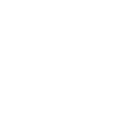
1 Writing Code About Your Infrastructure? That's a Losing Race 31:21
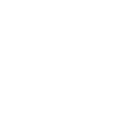
1 OpenTelemetry: What’s New with the 2nd Biggest CNCF Project? 30:14
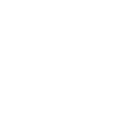
1 What’s Driving the Rising Cost of Observability? 24:55
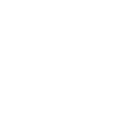
1 How Oracle Is Meeting the Infrastructure Needs of AI 27:28
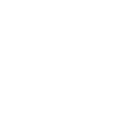
1 Arm: See a Demo About Migrating a x86-Based App to ARM64 21:28
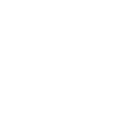
1 Heroku Moved Twelve-Factor Apps to Open Source. What’s Next? 22:54
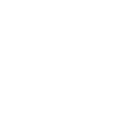
1 How Falco Brought Real-Time Observability to Infrastructure 19:27
Chào mừng bạn đến với Player FM!
Player FM đang quét trang web để tìm các podcast chất lượng cao cho bạn thưởng thức ngay bây giờ. Đây là ứng dụng podcast tốt nhất và hoạt động trên Android, iPhone và web. Đăng ký để đồng bộ các theo dõi trên tất cả thiết bị.