Chuyển sang chế độ ngoại tuyến với ứng dụng Player FM !
Evaluating Extrapolation Performance of Dense Retrieval: How does DR compare to cross encoders when it comes to generalization?
Manage episode 355037185 series 3446693
How much of the training and test sets in TREC or MS Marco overlap? Can we evaluate on different splits of the data to isolate the extrapolation performance?
In this episode of Neural Information Retrieval Talks, Andrew Yates and Sergi Castella i Sapé discuss the paper "Evaluating Extrapolation Performance of Dense Retrieval" byJingtao Zhan, Xiaohui Xie, Jiaxin Mao, Yiqun Liu, Min Zhang, and Shaoping Ma.
📄 Paper: https://arxiv.org/abs/2204.11447
❓ About MS Marco: https://microsoft.github.io/msmarco/
❓About TREC: https://trec.nist.gov/
🪃 Feedback form: https://scastella.typeform.com/to/rg7a5GfJ
Timestamps:
00:00 Introduction
01:08 Evaluation in Information Retrieval, why is it exciting
07:40 Extrapolation Performance in Dense Retrieval
10:30 Learning in High Dimension Always Amounts to Extrapolation
11:40 3 Research questions
16:18 Defining Train-Test label overlap: entity and query intent overlap
21:00 Train-test Overlap in existing benchmarks TREC
23:29 Resampling evaluation methods: constructing distinct train-test sets
25:37 Baselines and results: ColBERT, SPLADE
29:36 Table 6: interpolation vs. extrapolation performance in TREC
33:06 Table 7: interplation vs. extrapolation in MS Marco
35:55 Table 8: Comparing different DR training approaches
40:00 Research Question 1 resolved: cross encoders are more robust than dense retrieval in extrapolation
42:00 Extrapolation and Domain Transfer: BEIR benchmark.
44:46 Figure 2: correlation between extrapolation performance and domain transfer performance
48:35 Broad strokes takeaways from this work
52:30 Is there any intuition behind the results where Dense Retrieval generalizes worse than Cross Encoders?
56:14 Will this have an impact on the IR benchmarking culture?
57:40 Outro
Contact: castella@zeta-alpha.com
21 tập
Manage episode 355037185 series 3446693
How much of the training and test sets in TREC or MS Marco overlap? Can we evaluate on different splits of the data to isolate the extrapolation performance?
In this episode of Neural Information Retrieval Talks, Andrew Yates and Sergi Castella i Sapé discuss the paper "Evaluating Extrapolation Performance of Dense Retrieval" byJingtao Zhan, Xiaohui Xie, Jiaxin Mao, Yiqun Liu, Min Zhang, and Shaoping Ma.
📄 Paper: https://arxiv.org/abs/2204.11447
❓ About MS Marco: https://microsoft.github.io/msmarco/
❓About TREC: https://trec.nist.gov/
🪃 Feedback form: https://scastella.typeform.com/to/rg7a5GfJ
Timestamps:
00:00 Introduction
01:08 Evaluation in Information Retrieval, why is it exciting
07:40 Extrapolation Performance in Dense Retrieval
10:30 Learning in High Dimension Always Amounts to Extrapolation
11:40 3 Research questions
16:18 Defining Train-Test label overlap: entity and query intent overlap
21:00 Train-test Overlap in existing benchmarks TREC
23:29 Resampling evaluation methods: constructing distinct train-test sets
25:37 Baselines and results: ColBERT, SPLADE
29:36 Table 6: interpolation vs. extrapolation performance in TREC
33:06 Table 7: interplation vs. extrapolation in MS Marco
35:55 Table 8: Comparing different DR training approaches
40:00 Research Question 1 resolved: cross encoders are more robust than dense retrieval in extrapolation
42:00 Extrapolation and Domain Transfer: BEIR benchmark.
44:46 Figure 2: correlation between extrapolation performance and domain transfer performance
48:35 Broad strokes takeaways from this work
52:30 Is there any intuition behind the results where Dense Retrieval generalizes worse than Cross Encoders?
56:14 Will this have an impact on the IR benchmarking culture?
57:40 Outro
Contact: castella@zeta-alpha.com
21 tập
Todos os episódios
×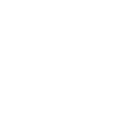
1 AGI vs ASI: The future of AI-supported decision making with Louis Rosenberg 54:42
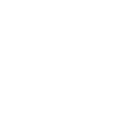
1 EXAONE 3.0: An Expert AI for Everyone (with Hyeongu Yun) 24:57
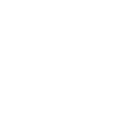
1 Zeta-Alpha-E5-Mistral: Finetuning LLMs for Retrieval (with Arthur Câmara) 19:35
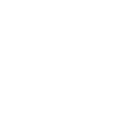
1 ColPali: Document Retrieval with Vision-Language Models only (with Manuel Faysse) 34:48
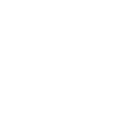
1 Using LLMs in Information Retrieval (w/ Ronak Pradeep) 22:15
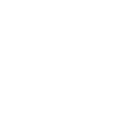
1 Designing Reliable AI Systems with DSPy (w/ Omar Khattab) 59:57
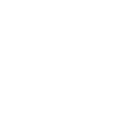
1 The Power of Noise (w/ Florin Cuconasu) 11:45
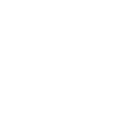
1 Benchmarking IR Models (w/ Nandan Thakur) 21:55
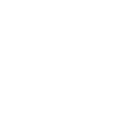
1 Baking the Future of Information Retrieval Models 27:05
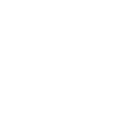
1 Hacking JIT Assembly to Build Exascale AI Infrastructure 38:04
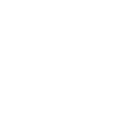
1 The Promise of Language Models for Search: Generative Information Retrieval 1:07:31
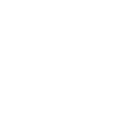
1 Task-aware Retrieval with Instructions 1:11:13
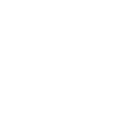
1 Generating Training Data with Large Language Models w/ Special Guest Marzieh Fadaee 1:16:14
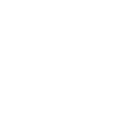
1 ColBERT + ColBERTv2: late interaction at a reasonable inference cost 57:30
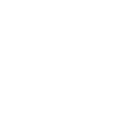
1 Evaluating Extrapolation Performance of Dense Retrieval: How does DR compare to cross encoders when it comes to generalization? 58:30

1 Open Pre-Trained Transformer Language Models (OPT): What does it take to train GPT-3? 47:12

1 Few-Shot Conversational Dense Retrieval (ConvDR) w/ special guest Antonios Krasakis 1:23:11

1 Transformer Memory as a Differentiable Search Index: memorizing thousands of random doc ids works!? 1:01:40

1 Learning to Retrieve Passages without Supervision: finally unsupervised Neural IR? 59:10

1 The Curse of Dense Low-Dimensional Information Retrieval for Large Index Sizes 54:13

1 Shallow Pooling for Sparse Labels: the shortcomings of MS MARCO 1:07:17
Chào mừng bạn đến với Player FM!
Player FM đang quét trang web để tìm các podcast chất lượng cao cho bạn thưởng thức ngay bây giờ. Đây là ứng dụng podcast tốt nhất và hoạt động trên Android, iPhone và web. Đăng ký để đồng bộ các theo dõi trên tất cả thiết bị.